In a recent study published in npj Digital Medicine, researchers evaluated the performance of a large language model (LLM) in phenotyping postpartum hemorrhage (PPH) patients using discharge notes.
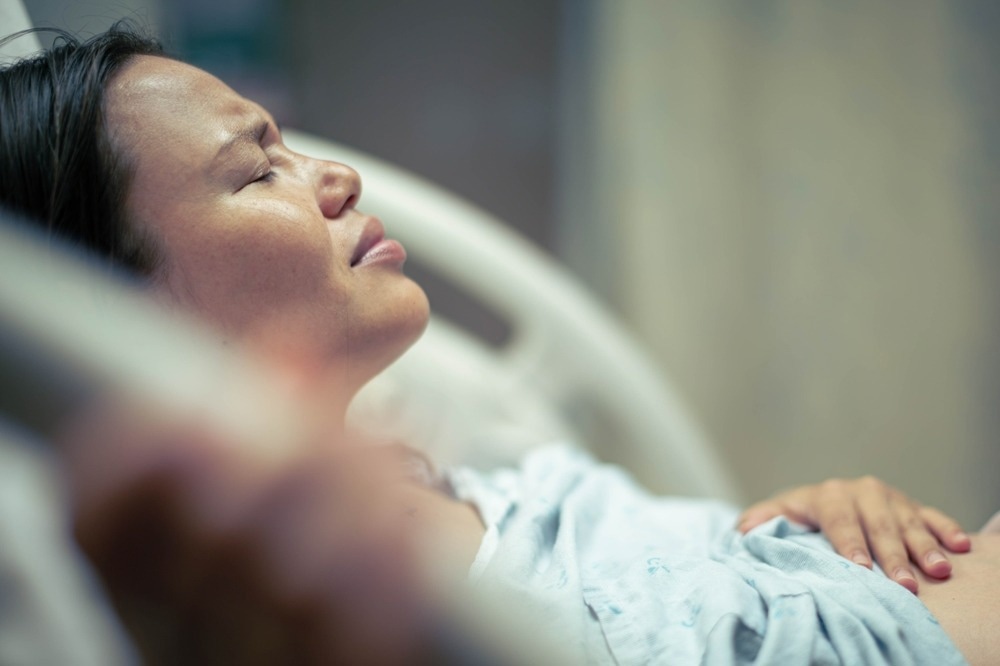
Background
Robust phenotyping is critical to research and clinical workflows, including diagnosis, clinical trial screening, novel phenotype discovery, quality improvement, comparative effectiveness research, and phenome- and genome-wide association studies. Adopting electronic health records (EHRs) has allowed for the development of digital phenotyping approaches.
Many digital phenotyping approaches leverage diagnosis codes or rules based on structured data. However, structured data often fails to capture the clinical narrative from EHR notes. Natural language processing (NLP) models have been increasingly used for multimodal phenotyping through automated extraction from unstructured notes.
Most NLP approaches are rules-based and rely on regular expressions, keywords, and other NLP tools. Recent advances in training LLMs allow the development of generalizable phenotypes without the need for annotated data. LLMs’ zero-shot capabilities present an opportunity to phenotype complex conditions using clinical notes.
The study and findings
In the present study, researchers developed an interpretable approach for phenotyping and subtyping of PPH cases by using the Flan-T5 LLM. They identified over 138,000 individuals with an obstetric encounter at the Mass General Brigham hospitals in Boston between 1998 and 2015. Discharge summaries were used for NLP-based phenotyping.
The team developed 24 PPH-related concepts and identified them in discharge notes by prompting the Flan-T5 model for two types of tasks – binary classification and text extraction. Identification of estimated blood loss was the text extraction task, whereas identification of other PPH-related concepts was a binary classification task. Fifty annotated notes were used to develop LLM prompts.
The performance of the model on 1,175 manually annotated discharge notes was evaluated. Flan-T5 NLP models were compared to regular expressions for each concept. The binary F1 score of the Flan-T5 model was ≥ 0.75 on 21 PPH concepts and > 0.9 on 12 concepts. The Flan-T5 model outperformed regular expressions for nine concepts.
Although regular expressions performed similarly to Flan-T5 on simpler tasks, the Flan-T5 model outperformed them on concepts expressed in clinical notes in different formats. False positives of the Flan-T5 model were primarily in notes with polysemy and semantically related concepts. For instance, notes containing dilation and curettage postpartum were often predicted as positive for manual placenta removal.
False negatives were due to concepts with misspellings and unusual abbreviations. While notes from a single hospital were used to develop prompts, Flan-T5 generalized well to notes from other hospitals. Additionally, when a sample of notes from 2015 to 2022 was evaluated, the binary F1 score of Flan-T5 was ≥ 0.75 on 14 concepts.
The model showed comparable results for most concepts in both settings. Next, the team used extracted concepts to identify PPH deliveries. Flan-T5 extracted delivery type and estimated blood loss from all notes. Notes were classified as describing PPH if the blood loss was more than 500 mL and 1000 mL for vaginal and cesarean deliveries, respectively.
The PPH phenotyping algorithm was evaluated by comparing Flan-T5 performance on 300 expert-annotated discharge summaries predicted by the model as deliveries with PPH. The positive predictive value of this algorithm was 0.95. PPH cases without delivery-related diagnosis codes were also identified with this NLP-based approach. Specifically, more than 47% of discharge summaries with PPH would not have been identified if diagnosis codes were used alone.
Finally, PPH concepts were extracted to classify PPH into subtypes. To this end, composite phenotypes were constructed for each subtype based on the presence of NLP-extracted PPH terms. The researchers found that approximately 30% of predicted PPH deliveries were due to uterine atony, 24% due to trauma, 27% due to retained products of conception, and 6% due to coagulation abnormalities.
Conclusions
Taken together, the study developed 24 PPH-related concepts and observed that the Flan-T5 model could extract most concepts, demonstrating high recall and precision. Moreover, the phenotyping algorithm identified significantly more PPH deliveries than would be identified using diagnosis codes alone.
Furthermore, these concepts can be used for interpretable and precise identification of PPH subtypes. The findings highlight how complex LLMs can be exploited to construct downstream interpretable models. This extract-then-phenotype approach allows easy validation of concepts and rapid phenotype definition updates.
Notably, recurrent or delayed PPH cases might have been missed as emphasis was placed on discharge summaries. Moreover, discharge notes may reflect institution-specific practices, and although the model was assessed for temporal generalizability, further validation is required across medical conditions.